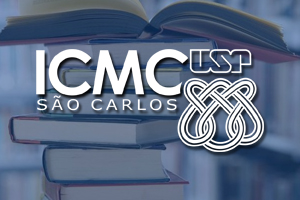
Resumo: TLatent variable models have many practical uses in modeling cross-sectional and longitudinal differences
in multi-dimensional datasets. For instance in psychometrics, latent variable models are used to model individual differences and account for measurement error in theoretical constructs like intelligence and
personality, and in econometrics, latent variable model are used to dynamically model econometric measures like stock wages over time. Estimation of these latent variable models has been dominated by
variations of maximum likelihood estimation, least squares estimation, and Bayesian estimation.
Although for continuous data, estimation is relatively fast and easily applied to high dimensional datasets, for discrete data, estimation is arguably more challenging. Therefore, recently interest has
grown in estimating latent variable models using neural networks. In this talk, we focus on specific neural network: The autoencoder. That is, we study how autoencoders from the field of deep learning
(Goodfellow et al., 2016) can be used to estimate latent variable models for discrete data from psychometrics in a fast and non-parametric way. Various challenges are addressed including parameter consistency, missing data, and robustness in the setup of the encoder.